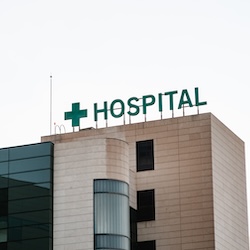
In the healthcare sector, revenue cycle management (RCM) is a process—patient registration through billing, collections, and reimbursement. Traditional RCM systems, while essential to profitability, are paper-based systems that are prone to inefficiency and mistake. Manual processes generate higher costs, delayed turnaround, and ultimately lost income opportunities. Generative AI is changing this narrative, in fact, this study suggests that 51% of healthcare providers are actively implementing generative AI for automating tasks and improving the accuracy of essential RCM functions. In the following sections, we’ll explore how this emerging technology is reshaping RCM and outline a step-by-step roadmap for healthcare organizations ready to implement AI-driven solutions.
Generative AI: A Revolutionary Force in RCM
Generative AI models are trained by the existing knowledge to create fresh content—words, pictures, or code—and not just forecast over labeled inputs. In RCM, these models are capable of automating clinical documentation, recommending proper billing codes, and filling out claim forms. Optimizing time-consuming procedures, generative AI de-emphasizes administrative work on top of the benefits of regulation compliance and up-to-date patient records. What sets generative AI apart from other machine learning is its ability to generate new content rather than classify or predict outcomes. In RCM, this means that the technology has the ability to actively generate billing documentation and interact with patients in real-time. As the report demonstrates, organizations can accelerate processes and reduce human error in a process that needs to be accurate. The constantly evolving healthcare regulation only adds to its complexity, and minimal patient participation normally leads to late payments. Generative AI solves these problems on several fronts. It reviews historical trends to underscore suspect claims prior to submission, reducing denial rates significantly. Intelligent chatbots and virtual assistants provide round-the-clock billing support, keeping patients informed of available payment options and clarifying unpaid balances. Bots automating the redundant tasks and increasing communication allow organizations to reallocate staff to more value-add activities, thus driving revenue and patient satisfaction.
Transforming RCM Workflows with Generative AI
Generative AI use is applicable to nearly all RCM aspects. In clinical documentation, AI apps crop patient encounter data and suggest appropriate billing codes, removing the guesswork usually leading to claim delays or denial. In claims processing, generative AI automatically fills in forms and scans them for common errors, finding inconsistencies long prior to payment cycle impact. Patient engagement is also a factor to consider. Virtual assistants driven by AI enable patients to query their bills at any time, with real-time answers and descriptions of payment plans. This kind of transparency promotes trust and facilitates the revenue collection process.
The Advantages of Generative AI in RCM
Generative AI has some considerable benefits, starting with reduced operational expenses.
- Organizations can automate repetitive work, lower the risk of denial of claims, and allow their team members to concentrate on high-value work.
- Precision is also very critical; automated data entry, as well as proper documentation, makes it simpler for organizations to get through audits and penalties.
- Patient satisfaction is enhanced through more open and faster billing communication. By providing easily viewable information about their bills, patients are more likely to pay on time and have a overall good experience—an objective that aligns with the value-based care path of the industry.
- Finally, generative AI is extremely scalable. When healthcare practitioners increase the number of patients on their rolls, the RCM systems built around AI can handle more data without compromising on speed or consistency.
A Roadmap for Implementation
Prior to installing generative AI, there needs to be a thorough review of current procedures.
- Identify key pain points such as denial rates or patient response times and then set concrete goals—whether reducing denial rates, maximizing cash flow, or improving patient engagement.
- Selecting the appropriate AI platform that can achieve these goals is also important. Seek solutions that are founded on your precise needs, be it predictive analytics, claims drafting, or clinical documentation.
- Get your data right and structured to enable AI models to generate rational insights. Phase in AI capabilities: start with a limited scope (e.g., claims processing) to learn, prove ROI, and only subsequently expand to broader workflows.
- Thorough employee training and buy-in are essential—when employees understand that AI complements, rather than displaces, their work, companies can more easily achieve early wins that drive subsequent momentum.
Overcoming Implementation Challenges
The deployment of generative AI into RCM comes with some potential challenges, that of data security and privacy. Healthcare organizations must remain in compliance with regulations like HIPAA by employing robust security controls to protect confidential patient information. Resistance to change can also be a hindrance. Employees may resist new technology because they fear losing their jobs or not knowing the new workflows. Open communication, transparency, and ongoing training build trust in AI as a productivity tool, not a substitute for human abilities. While the upfront investment will be expensive, the long-term efficiency and revenue capture advantages typically pay for the expense.
Real-World Success Stories
Several large US healthcare systems have already applied generative AI to claims processing and seen much lower denial rates. As per the HFMA report, medical centers reduced their denials by 22% within six months, equating to millions of dollars of harvested revenue. In a different situation, a medium-sized provider used co-pilots for analyzing and processing claims using EOBs and improved 60% in processing time without human touch. As per the article, Doctors and Nurses in Taiwan spend less time in writing patient medical and information reports. These findings not only moved the bottom line but also improved patient loyalty by simplifying and becoming more transparent when communicating. Generative AI is in progress and its use in healthcare RCM will keep increasing. Some of the potential is for real-time claims processing with reduced human touch, dynamic pricing schemes using predictive analytics, and advanced fraud detection tools that can pick up anomalies in billing behavior. Early adopters with generative AI will be able to ride an increasingly complex financial environment while improving operational efficiency and patient outcomes.
Conclusion
Generative AI offers a groundbreaking opportunity at tipping the healthcare revenue cycle balance, turning laborious and error-prone activities into effective procedures that generate better financial outcomes as well as patient satisfaction. Successful deployment begins with clearly outlined goals and phased implementation supported by sound data governance and ongoing employee education. By embracing AI-driven solutions now, healthcare organizations can future-proof their RCM systems and enhance the quality of care they provide—while achieving higher efficiency and more sustainable revenue streams.
About the Author
Loganandh Natarajan is a seasoned cloud and data professional with over 20 years of experience in the Information Technology industry, specializing in Cloud/Data Architecture and Artificial Intelligence. He currently serves at Optum, a division of UnitedHealth Group, a Fortune 5 company. In his role, Loganandh guides high-performing teams in designing advanced analytics frameworks, implementing scalable cloud infrastructures, and driving AI-powered initiatives to streamline operations and enable data-driven decision-making. Follow Loganandh at: LinkedIn.
Disclaimer: The author is completely responsible for the content of this article. The opinions expressed are their own and do not represent IEEE’s position nor that of the Computer Society nor its Leadership.